Transparency and control for recommender systems
Recommendation systems have become ubiquitous in the digital world, spanning from social media content to target advertising and product and service recommendations. Consumers are increasingly demanding transparency on the use of these algorithms and, more recently regulations, such as the EU Digital Services Act, are being put forth.
Learn how Deeploy can be leveraged to deliver this transparency to consumers and regulators, allowing organizations to deploy and benefit from the use of AI models while enhancing consumer trust, experience, and satisfaction.
Organizations using Deeploy
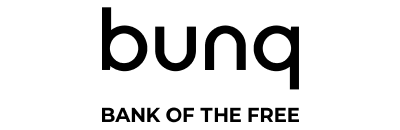
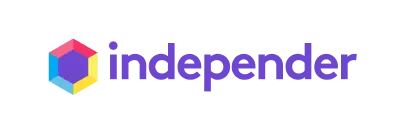
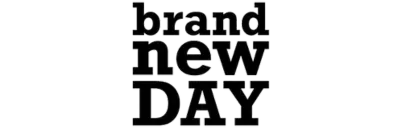
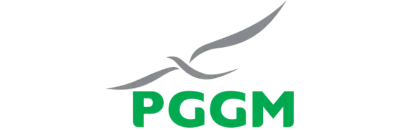
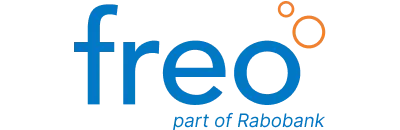
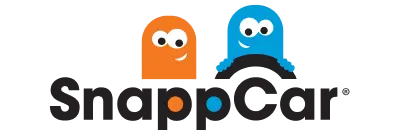
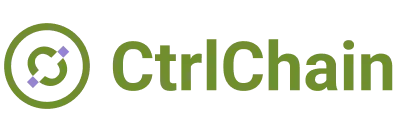
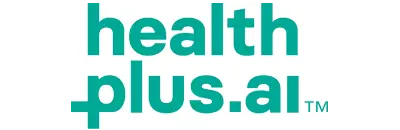
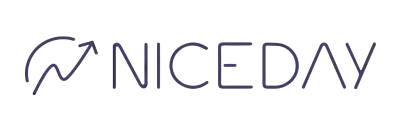
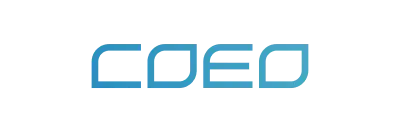
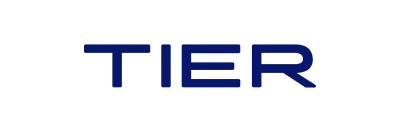
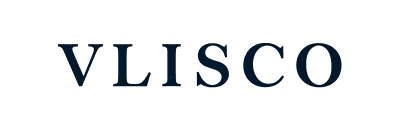
Increase consumer trust
Make recommendation engines transparent
Allowing users to clearly understand items like their news feeds or product recommendations is vital to reach fair online business and increases consumer confidence. This is where explainable AI comes into play. In practice, explainability refers to the ability of platforms to provide clear and understandable explanations to end users about why a particular recommendation, such as a product, news article, or video, was made.
When deploying a model on Deeploy, data teams can do so using a variety of explainability techniques as well as custom explainers. This ensures that every recommendation the consumer receives is accompanied by the why behind that recommendation.
In the background, the explainability method determines what type of information has the largest weight, and returns that weight as output. That output is translated to a UI element, as shared in the visual above.
By incorporating explainability methods, platforms can bridge the gap between complex algorithmic processes and user comprehension, helping users make informed decisions and maintain trust in the platform’s recommendations while ensuring compliance with regulations.
Allow for user control and feedback
Besides being shown the reasoning behind recommendations, another way to foster consumer trust and empowerment is to give users control over the recommendations they receive as well as the option to give feedback on the quality of recommendations.
Besides giving users more control, implementing a feedback loop also offers an opportunity to improve model performance and accuracy. The feedback given by users can then be fed back into Deeploy where it is translated into the disagreement ratio metric. Monitoring this metric can help data teams determine model effectiveness and identify areas for improvement.
Comply with regulations
The rules specified in the Digital Services Act demand that any meaningful information about the main parameters used to determine that a specific advertisement was shown to that specific user be made clear. Likewise, platforms that include recommender systems must explain the main parameters used in their recommender systems. Read more on the requirements of the DSA here.
Maintain transparent operations
Besides directly showing consumers the reasoning behind recommendations, in Deeploy, all model predictions/recommendations and their accompanied explanations can be traced back and reproduced, creating a full audit trail over model decisions. The predictions log can also be filtered for specific periods, aiding in pinpointing any potential issues or incidents.
Stay in control of all use cases
The growing number of models scattered across organizations leads to an increasing lack of control which introduces governance and compliance risks. Deeploy enables teams to deploy, serve, monitor, and manage AI models in one single platform, allowing for easier oversight while saving time and resources.
Apart from combining all necessary monitoring metrics into one platform, Deeploy also allows alerts to be set for all metrics, allowing teams swift action in case of model degradation.
Keep model information & records readily available
Regulations also underscore the need for information about each AI application to be easily available and for all events around the models to be logged for future consultation.
Deeploy facilitates these requirements by allowing teams to create and store model cards, with information on model capabilities, intended use, performance characteristics, and limitations. Additionally, all events for every model deployment are automatically recorded and stored, creating a full model registry that can be consulted when needed.
Stay on top of compliance processes
When deploying AI use cases, organizations must be aware of an increasing number of regulations and requirements. To facilitate the work and communication between data and compliance teams, Deeploy offers standard and customizable compliance templates, enabling teams to verify that compliance requirements are fulfilled for every AI application within the organization.
While standard templates offer general guidance on high-level regulation, the feature on custom compliance documentation, allows teams to upload checklists tailored to fit specific requirements and policies of the organization, as, the Digital Services Act.
How to get started with Deeploy
Would you like to learn more about how you can take your first steps with Deeploy? Let one of our experts walk you through the platform and how it can be leveraged for your specific concerns or start with a trial of our SaaS solution.