Compliant AI in Insurance
AI is improving all aspects of the insurance sector, spanning distribution, underwriting, dynamic pricing, claims management, and customer service. Yet, not only do these advances face heavy scrutiny from regulators but the mishandling of AI can lead to substantial reputational harm. Insurers who prioritize transparency and reliability will establish long-term security, gain competitive advantage, and stay ahead of regulatory requirements.
Learn more about Deeploy and how leading organizations, such as Independer, leverage the platform to stay in control and maintain transparent AI operations, mitigating risks and staying ahead of competition.
Financials using Deeploy
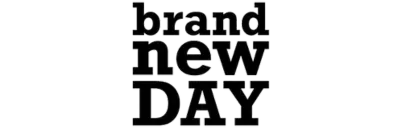
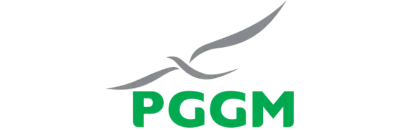
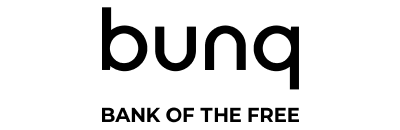
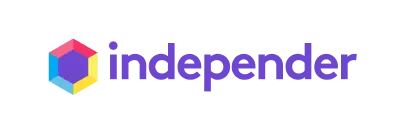
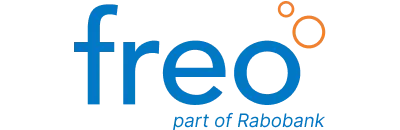
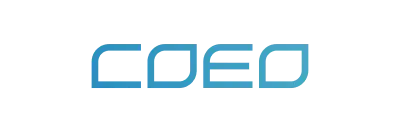
Establish trust with consumers
Reputational damage from the misuse or untransparent use of AI can quickly cause significant customer churn. To avoid this, insurers must be transparent about their AI use cases and take a responsible approach to AI, reassuring and creating trust with customers.
Ensure model transparency & explainability
The complexity of AI models, in particular, can create concern among customers, who might have doubts on how insurers use AI models to provide recommendations or offerings. It is then important that models are fully transparent and explainable as well as understandale to consumers.
Deeploy is tailored for explainability. When deploying a model on Deeploy, data teams can do so using a variety of standard explainability techniques as well as custom explainers. This can then be translated onto a front end where consumers can be shown the reasoning behind any AI recommendation or offering they might receive.
The same explainability applies internally to employers and stakeholders that receive decision support from AI models. This increases trust and adoption and allows organizations to maintain transparent operation of models from the get-go.
Maintain human oversight
Having expert employees be able to overlook and overrule model decisions acts as a safeguard against potential errors and biases and increases trust. Deeploy allows for end users of models to evaluate and give feedback on model decisions. This feedback can then be fed back into Deeploy where it is translated into the disagreement ratio metric.
The accuracy of a model can then be evaluated by comparing predicted outcomes to real-world observations provided by feedback. This helps teams determine model effectiveness and identify areas for improvement.
Moreover, it is also possible to set alerts for the disagreement ratio, meaning teams get notified when the disagreement ratio gets too high, which can inform when to perform a model update.
Monitor performance & accuracy
Guaranteeing that AI applications are solid and continue to perform well throughout their lifecycle is another vital piece to achieving trust. To ensure this, models must be monitored over time for traffic, errors, performance, and drift, among other metrics.
Deeploy combines all necessary monitoring metrics into one platform, facilitating the work of data teams. Moreover, alerts can also be set for any metrics, allowing teams swift action in case of model degradation.
Comply & report to regulators
Insurers are under increasing pressure from regulators regarding AI use. Namely, recent legislation, such as the EU AI Act, now define AI systems intended to be used for risk assessment and pricing in the case of life and health insurance to be high risk and thus subject to strict transparency, security, and fairness requirements.
Maintain & show transparent operations
Model operations must then be transparent and well-documented to allow insurers to report to regulators with full transparency and demonstrate AI is being implemented appropriately. In Deeploy, all model predictions/recommendations and their accompanied explanations can be traced back and reproduced, creating a full audit trail over model decisions. The predictions log can also be filtered for specific periods, aiding in pinpointing any potential issues or incidents.
Keep model documentation & records readily available
Insurers must make sure that information regarding each AI application is easily available and that all events around the deployment are logged. Deeploy facilitates these requirements by allowing teams to create and store model cards, with information on model capabilities, intended use, performance characteristics, and limitations. Additionally, all events for every deployment are automatically recorded and stored, creating a full model registry that can be consulted when needed.
Ensure clear oversight and accountability
The variety of use cases in production can easily make insurers lose oversight. This makes tasks like monitoring performance inefficient while leading to increased governance risks.
Ensuring clear oversight and accountability over running models not only leads to better AI governance but is also a key requirement of compliance with regulations. Deeploy enables teams to deploy, serve, monitor, and manage AI models in one single platform, providing a unified view over all running models.
Moreover, it is possible to assign owners to specific models, who are then responsible for maintaining control and reporting its ongoing operation. Furthermore, a clear distinction between teams and workspaces helps ensure the right oversight over different AI applications within the same organization. Teams can be created to differentiate between departments and types of applications, while workspaces can be used to further drill down model access.
Stay on top of compliance
To ease compliance, it is also useful for teams to keep track of progress in fulfilling requirements. Deeploy offers both standard and customizable compliance templates, enabling teams to verify that compliance requirements are fulfilled for every AI application within the organization.
While standard templates offer general guidance on high-level regulation, the feature on custom compliance documentation, allows teams to upload checklists tailored to fit specific requirements and policies of the organization.
How to get started with Deeploy
Would you like to learn more about how you can take your first steps with Deeploy? Let one of our experts walk you through the platform and how it can be leveraged for your specific concerns or start with a trial of our SaaS solution.