Compliant AI Transaction Monitoring
Using AI for transaction monitoring can speed up processes by 90% compared to traditional rule-based approaches. However, a lack of oversight over running models, regulatory requirements, and a lack of trust from end users and stakeholders is hindering implementation in financial institutions.
Leveraging explainability and oversight over running models is the key to successful implementation, giving financial institutions that apply this a head start on competitors and a strong foundation to avoid hefty regulatory fines. Read on for how bunq and other financials have used Deeploy to meet requirements of AI usage in banking and ensure successful productionalization.
Financials using Deeploy
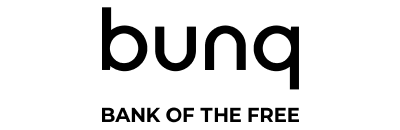
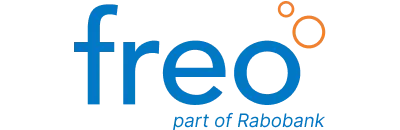
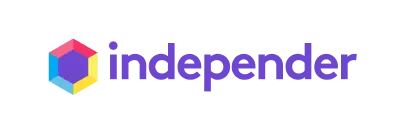
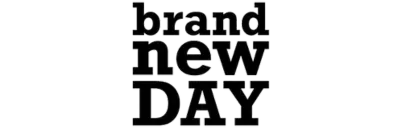
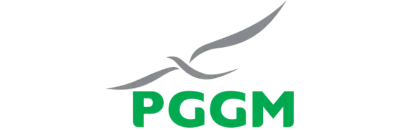
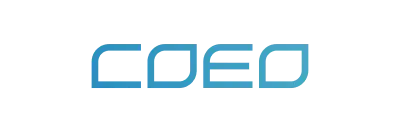
How AI revolutionizes transaction monitoring
Detection of Sophisticated Schemes
AI transaction monitoring systems outperform traditional methods by continuously improving detection through feedback, adapting to new fraud patterns autonomously and understanding evolving threats better.
Efficiency at handling Big Data
AI algorithms excel at real-time analysis of large transaction volumes, swiftly identifying potential threats like large transfers or unusual customer behavior, which traditional systems may struggle to handle effectively due to increasing data volume, velocity, and variety.
Lower False Positive Rates
AI reduces the number of false positives compared to traditional systems by leveraging extensive historical data and context, allowing it to distinguish between genuine and suspicious transactions more accurately. This streamlines investigations and optimizes resource allocation.
Less Resource Intensive & More Scalable
Rule-based systems demand laborious and expensive upkeep. AI, however, offers unmatched scalability, efficiently managing vast data volumes with minimal overhead costs.
Successfully implementing AI in transaction monitoring
AI models for transaction monitoring assess the probability of fraud for each transaction based on historical patterns, profile information, and transaction details. When a transaction is flagged, an investigation by a Compliance Operations Specialist (ComOps) follows. This investigation can lead to a hit and report of the user to the Financial Intelligence Unit (FIU), a hit but with no reporting of the user or complete clearing of the transaction.
While promising, institutions run into issues of lack of oversight over running models, regulatory requirements, and a lack of trust in AI decisions from ComOps and stakeholders.
How does Deeploy solve these challenges?
Centralized model deployment & monitoring
Often, models are scattered across teams and silos with no real oversight. This lack of oversight raises critical questions: Which models are operational? What are their objectives? How well are they performing? Is there a decline in accuracy?
This fragmentation of production hinders the effective and efficient implementation of models for transaction monitoring. Additionally, financial regulators also expect institutions to manage AI models effectively. This includes validating the accuracy, fairness, and reliability of AI algorithms used in transaction monitoring and implementing appropriate governance and oversight mechanisms.
Deeploy offers a solution by centralizing the deployment of all models onto a single platform, where they can be organized by teams and further categorized into workspaces.
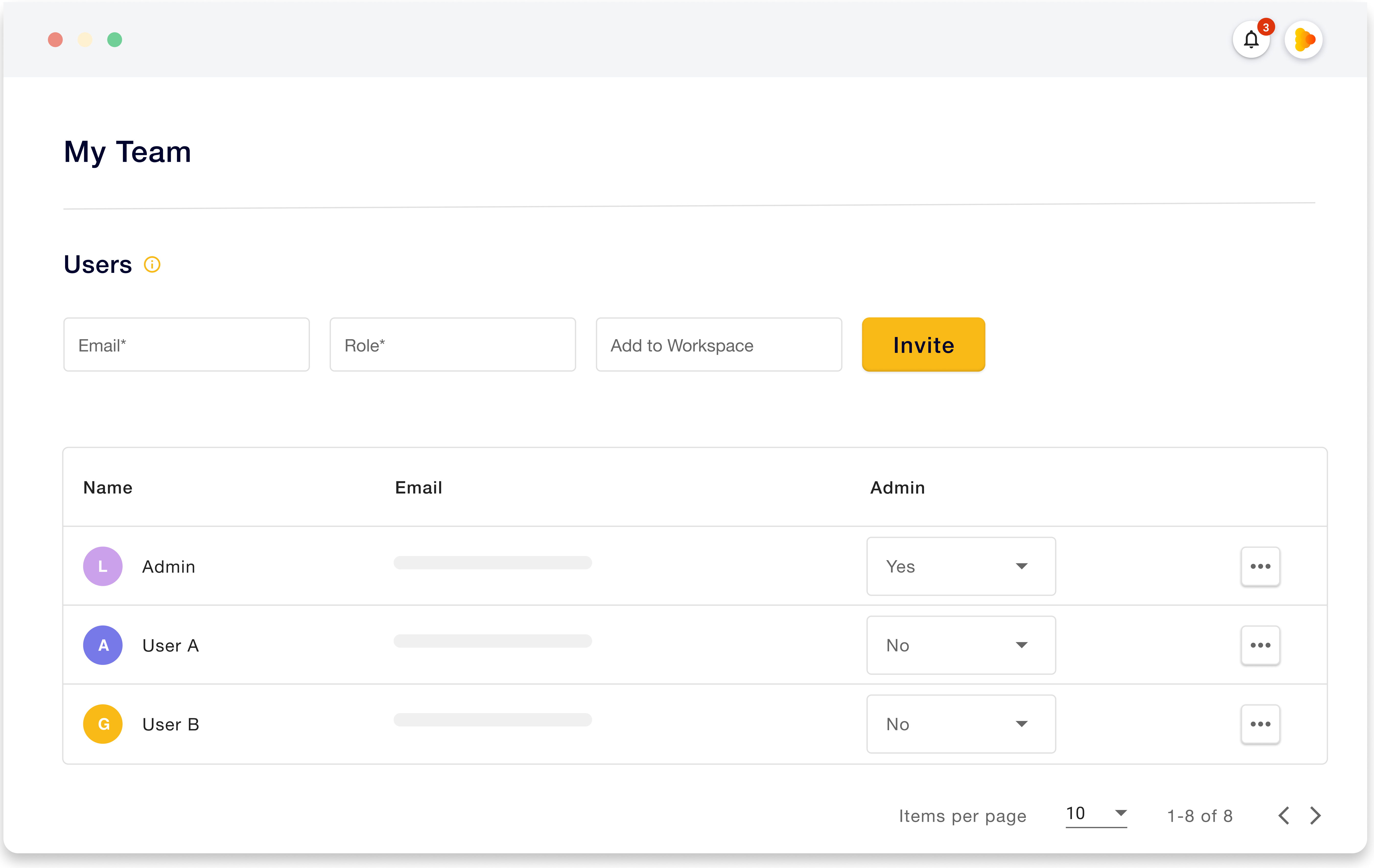
Through an intuitive interface, data science teams can then monitor running models across vital metrics and set personalized alerts to quickly address any degradation or anomalies. Furthermore, seamless integration with leading MLOps platforms such as Sagemaker, Azure ML, and MLFlow facilitates adoption for any team.
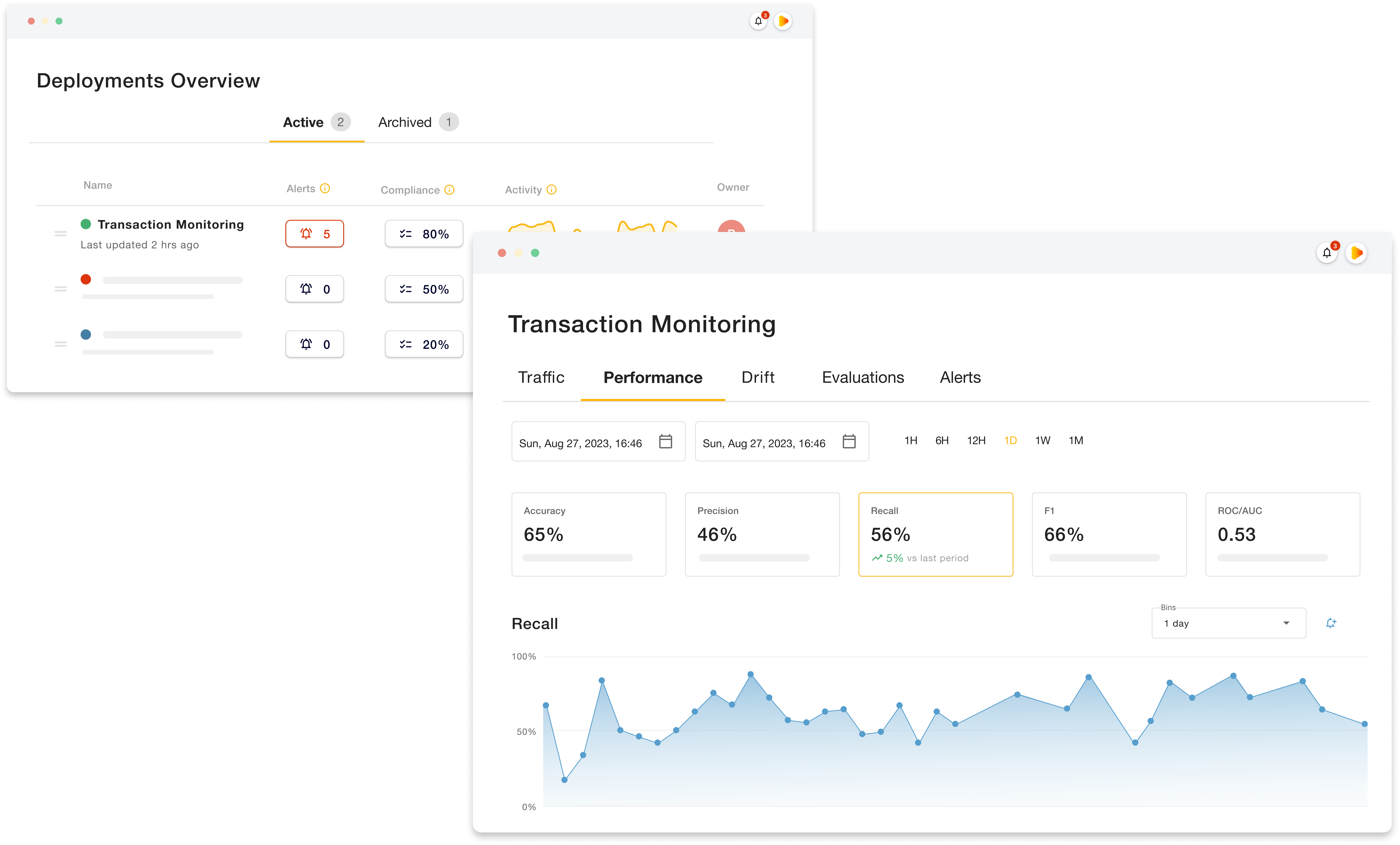
Deeploy also has all the necessary safety features in place to ensure sensitive data stays in control of the institution and model access is well managed trough various levels of user authorization and use of credentials.
Tailored explainability & feedback loops
Employees and stakeholders may be hesitant to fully trust AI-based transaction monitoring systems. AI models can be highly complex, making it challenging to understand how they arrive at certain decisions or predictions. Lack of interpretability can also hinder regulatory compliance efforts as authorities often require explanations for flagged transactions.
In addition, AI models can also inadvertently perpetuate biases present in the data used for training. Biased transaction monitoring may lead to unfair treatment of certain individuals or groups, which can result in legal and reputational risks for financials.
Deeploy makes it easy for teams to deploy models along with explainers, which can then be connected to an interface that allows ComOps agents to see which features affected a particular transaction flagging.
ComOps agents can overrule model decisions as well as provide feedback on the decision and the usefulness of the explanation provided. This feedback can then be used in the so-called human feedback loop which allows for continuous model improvement.
On Deeploy, data science teams can then monitor the percentage of overruled decisions trough the disagreement ratio metric. When big increases in this metric are detected, there might be a reason to retrain models and explainers. Teams can also set alerts for this metric, allowing for a greater manageability and oversight.

Traceability, reproducibility & compliance
Since AI systems require access to sensitive financial data to effectively monitor transactions, compliance requirements are a substantial concern for stakeholders.
Regulatory compliance often requires maintaining detailed audit trails and documentation of transaction monitoring activities. Financial institutions must ensure that their AI systems can provide sufficient documentation to demonstrate compliance with regulatory requirements. AI systems used in transaction monitoring must also be capable of generating accurate reports in compliance with regulatory requirements. Deeploy offers comprehensive auto recording of all deployment updates and events and the ability to traceback & reproduce all predictions/decisions.
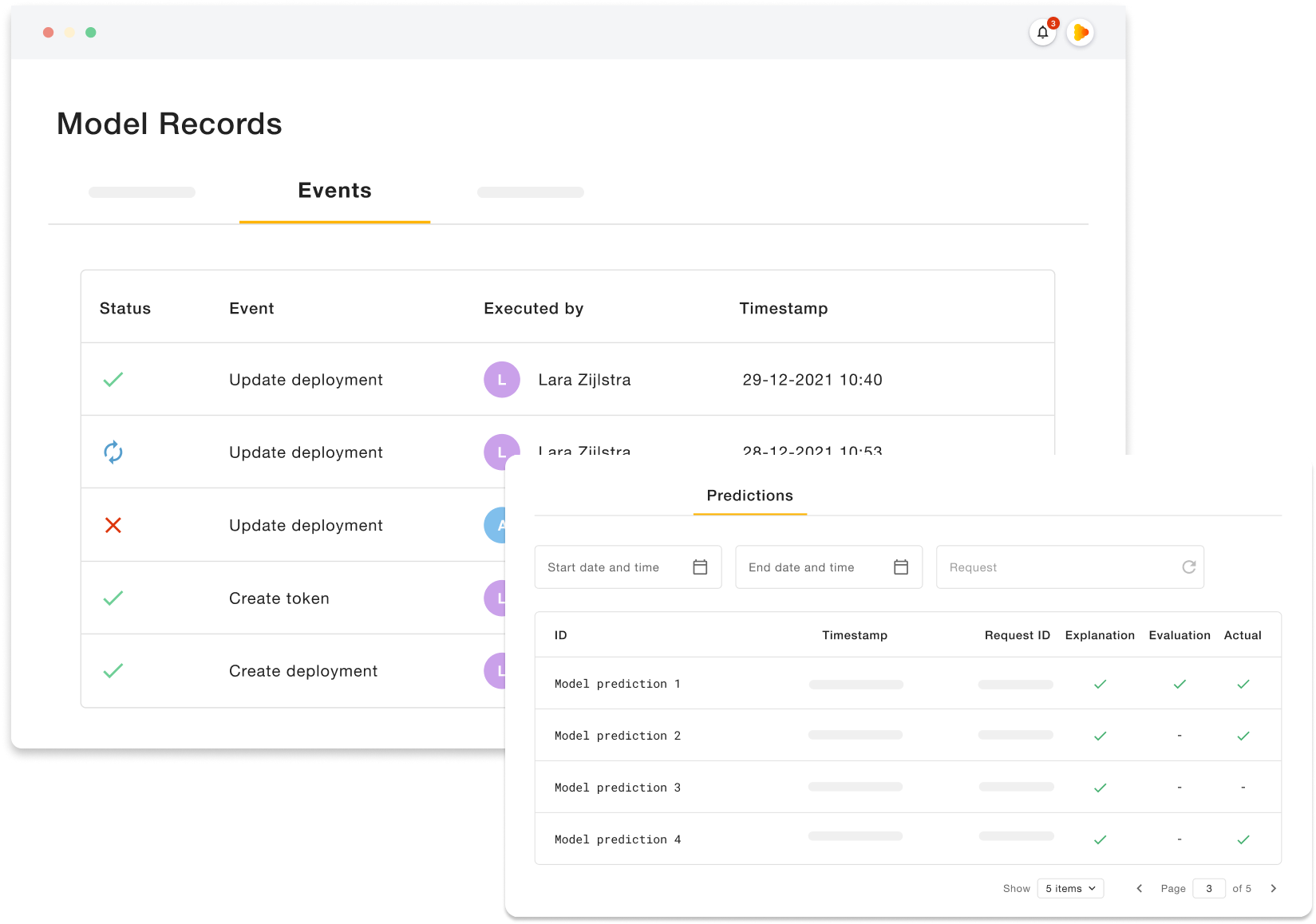
In addition to this, evolving regulations on the use of AI, such as the EU AI Act, intensify scrutiny of the deployment of AI systems in high-risk scenarios. Deeploy’s platform is built with these regulations in mind, ensuring an appropriate level of transparency and human oversight over AI models and offering dedicated compliance features.
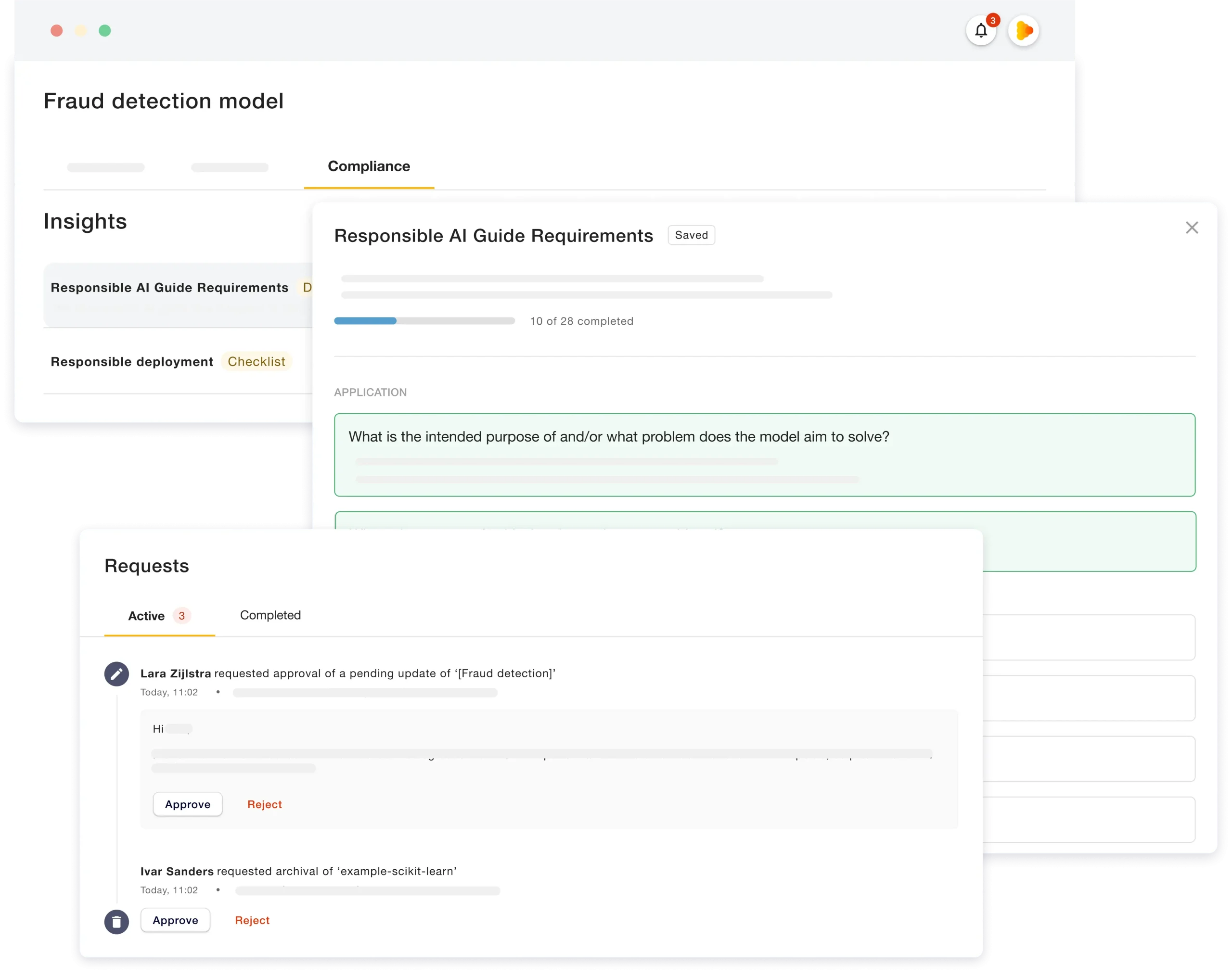
How Deeploy fits in your architecture
Deeploy empowers financial institutions to deploy high-risk AI systems while upholding transparency, control, and compliance standards.

By leveraging Deeploy, organizations can significantly reduce the burden on their data teams, saving valuable time spent on maintenance tasks and facilitating the monitoring process. Moreover, a full stack of integrations makes adoption seamless, avoiding costly implementation time.
How to get started with Deeploy
Would you like to learn more about how you can take your first steps with Deeploy? Let one of our experts walk you through the platform and how it can be leveraged for your specific concerns or start with a trial of our SaaS solution.